This article discusses automatically scoring film analysis essays. Here are links to other automated evaluations on the Virtual Writing Tutor.
Lately, I have been developing automatic essay evaluations for IELTS and now for college-level academic writing. I sent an email to colleagues in my department to share my progress, and one colleague wrote back to share his concerns about my new automatically self-scoring film analysis essay writing assignment.
I had shared a sample of a film analysis essay and a link to the academic essay writing test. He had tried the test with the sample essay, and though impressed, he was concerned about the extent to which we can automate essay evaluations.
There are lots of variables in such texts that, I believe, cannot be analyzed in a systematic way. And those variables are often what separates a great essay from a passable one. A grabber is not just a question. It has to be stimulating. A thesis has to be precise and thought provoking. The topic sentences have to be directly linked to the thesis as well as provide further insight. Can a computer truly give relevant feedback on whether or not something is stimulating, precise, thought-provoking and providing further insight on a previous idea?
An ESL teacher on the VWT’s new automatic essay evaluator
College teachers are required to assign a value to a student’s text, but if we are not assigning a value based on observable, measurable criteria, what are we scoring? Are teachers scoring essays based on their own subjective reaction to an essay? Or are we looking for features that indicate achievement, features that indicate the student has learned how to answer a question in-depth and in a systematic way? If teachers are just feeling their way to a score, woe to the poor student whose academic advancement depends upon such a non-rational scoring rubric.
Can a computer give relevant feedback?
Even so, I share all my colleagues doubts about brute force calculations of good writing. Surely, narrow artificial intelligence that calculates scores based on lists of structural features, lexical items, and grammatical error patterns will miss the value of a meaningful expression of nuanced human intelligence.
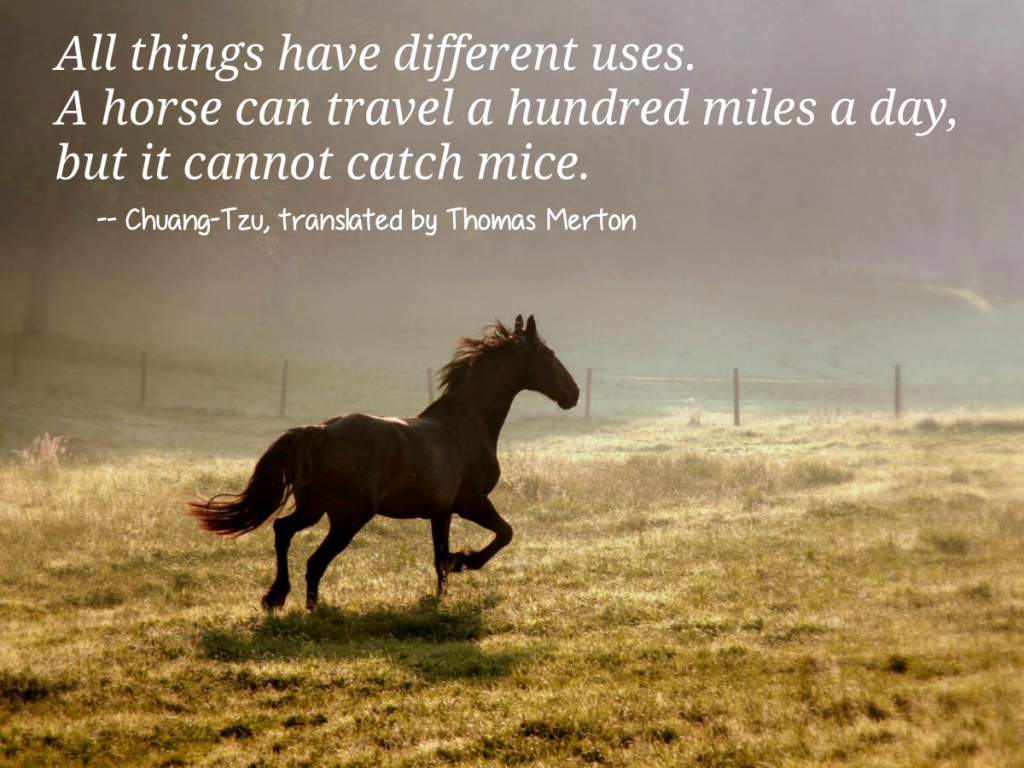
Can computers provide feedback of real value? Is automatic essay scoring just an impossible dream?
Doubts about what computers can do remind me of an observation made by the ancient Taoist philosopher Chuang-Tzu.
“All things have different uses. A horse can travel a hundred miles a day, but it cannot catch mice.”
Chuang-Tzu, translated by Thomas Merton
I read those words back when I was a college student. They have stuck with me for 30+ years. Is it wise to determine the value of a horse by what it can’t do? It seems like the kind of argument one might make at the market while trying to negotiate a lower price.
Let me put it another way. Oisin Woods, a German teacher and colleague in our Modern Languages Department at Ahuntsic College, told me something I won’t soon forget. He said, “Of course computers can’t do everything a teacher can do, but let’s not make perfection the enemy of the good.”
Let’s not make perfection the enemy of the good.
Oisin Woods
These two epigrams seem to point in the same direction. Focusing on what a computer cannot do distracts us from what it does well. It is like complaining that horse cannot catch mice. What’s more, if we make pedagogical perfection our only goal instead of energetic strides toward better feedback and better pedagogy, we’ll get neither perfection nor the progress we crave.
Rather, it is better to explore what machines can do in the service of good pedagogy. Machines can count, they can match patterns, and they can respond to errors in seconds. Humans can count and match and correct, but much more slowly. More importantly, humans understand and reflect. We should be allies in the provision of feedback, don’t you think?
After all, feedback works best when it is just-in-time, just-for-me, just-about-this-task, and just-what-I-need-to-improve. If we can use technology to ensure faster, personalized, and more focused feedback, that has got to be a good thing.
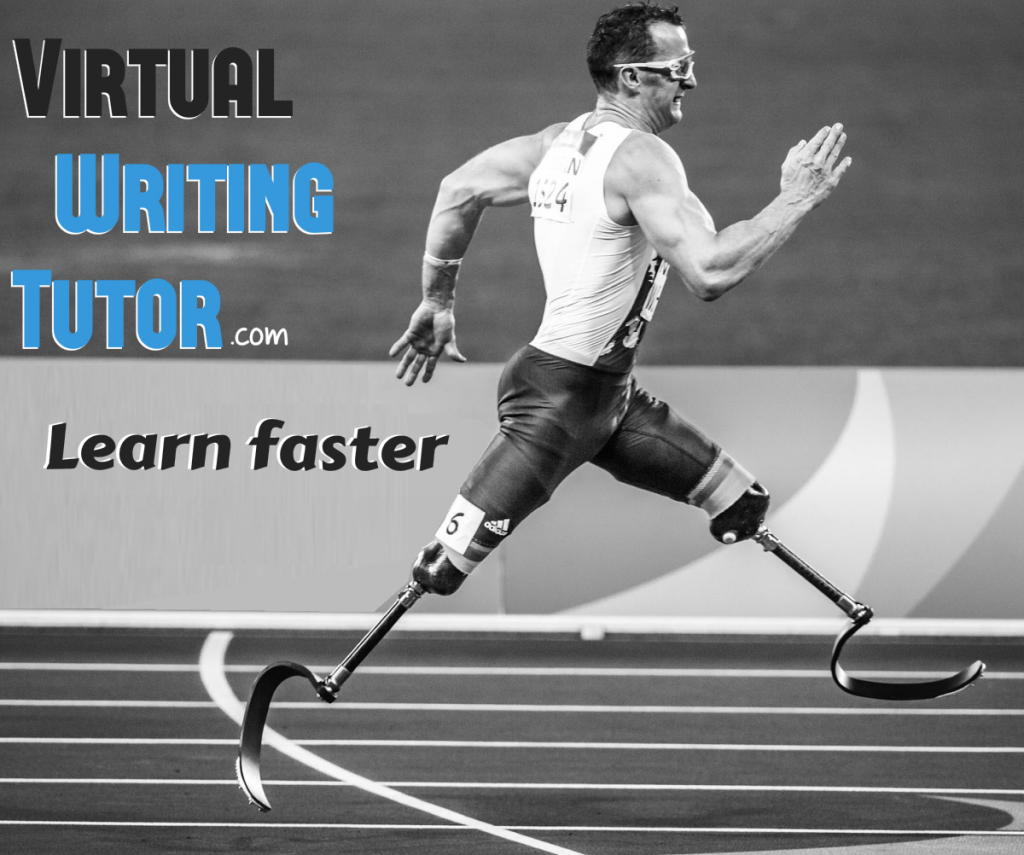
A research question and a hypothesis
The practical pedagogical question I ask myself these days is this, “Can narrow artificial intelligence provide useful formative feedback to learners and help teachers score essays more reliably?” The answer seems to be, on balance, “yes.”
Automatic scoring and feedback will help students become better writers and help teachers evaluate essays more reliably.
My current hypothesis
A null hypothesis
Let’s see if there is any evidence in the research literature to support a null hypothesis. Science doesn’t try to prove a point that can just as easily be disproved. The case is not as open and shut as technophiles might have hoped.
- Computers can be fooled by clever nonsense (Monaghan & Bridgeman, 2005).
- Brilliant non-conformist writing will score lower because it is eccentric (Monaghan & Bridgeman, 2005).
- Automatic scoring of complex argument essays is less reliable than of inherently less complex opinion essays (0.76 vs. 0.81) (Bridgeman, 2004). The difference in reliability of 0.05 is small but significant.
These seem to fit with concerns that some elements of meaningful essays cannot be analyzed programmatically in an effective way. Computers do not construct and test a world model in their imaginations the way humans do when they read. Text coherence will thus remain elusive for non-conscious computerized agents because pattern-matching is not reading in our sense of the word.
So what, you say? Horses can’t catch mice! Let’s consider another objection to automatic evaluation.
My colleague asked, “Can a computer truly give relevant feedback on whether or not something is ‘stimulating’, ‘precise’, ‘thought-provoking’ and ‘providing further insight on a previous idea’?” The answer is probably, “no.” I certainly have my doubts, but I also have my doubts that a teacher can explain the mechanics of why a sentence stimulates or provokes thought. All I have ever been able to do in these areas of writing is dramatize the presence of a reader by indicating when a sentence stimulates or provokes me, often with cryptic or terse comments in the margin: Wow! Nice! Interesting! Provocative!
A reasonable hypothesis
Turning to possibilities and evidence for my hypothesis that automatic scoring and feedback could help students become better writers and help teachers evaluate essays, here is what I have observed and what I have read.
Faster feedback
At my college, we see students once a week, and teachers regularly take two weeks to provide feedback on a first draft. The VWT takes two seconds. That’s 20,160 minutes versus 2 minutes, which is a million times faster.
Workload reduction
Teachers limit the number of essays students write because of the impact of corrections on the teacher’s workload. With 150 student essays to grade, if a teacher spends just 10 minutes grading an essay, one essay assignment adds 25 hours of non-stop grading and correction work to a teacher’s workload. Necessity has made us put time-resource limitations ahead of the pedagogical goal of maximizing meaningful practice with a focus on form. In other words, there are not enough teachers to provide all of the feedback students require (Monaghan & Bridgeman, 2004).
Latent essay feature analysis
Automatically comparing multiple features of a student’s essay to an ideal essay was found to provide useful formative feedback to students (Foltz et al., 1999). This is interesting! Students want to improve, and one way to measure essay writing skill is by comparing what the student has written to a model essay to show the student how to improve further. By abstracting the features of the model essay and comparing the studentès essay to it programmatically, we can show the student where he or she has diverged from the ideal.
Reliability
Automatic scores using grammar, topic, discourse features, and sentiment analysis are very highly correlated to expert human scores (Farra et al., 2015). That’s encouraging because the reliability of a teacher’s ratings of student essays declines with fatigue. Machines don’t tire and can evaluate essays consistently.
Researchers found that using one essay task and one human evaluator to measure achievement produced unreliable scores (Brendland et al., 2004), and yet that is what we do at finals every semester.
A computer rating combined with 1 human rating was found to be more reliable than the combination of scores by 2 human raters (Bridgeman, 2004). Computer-assisted scoring is more reliable than both exclusive computer scoring and exclusive human scoring. Humans diverge too much in their judgements.
All that said, I think that there is evidence that a fast and free source of computerized formative feedback available online 24 hours a day is likely to help students improve their writing and their self-assessments of their writing.
Two ways to improve performance dramatically
John Hattie (2009) found in his meta-analysis of 800+ meta-analyses that the most effective thing students can do to improve their own performance is to openly declare to the class what score they expect to achieve on an upcoming evaluation. Why? Because making a prediction about your next score is akin to setting a goal to achieve that score.
Curiously, minority group students tend to inflate their predictions. In contrast, girls tend to minimize their predictions. However, practice tests make all students more reliable at predicting their own performance. This is important because when students get better at predicting their performance, they need less feedback from the teacher. They already know how well they are doing.
The second most effective intervention that teachers can use to maximize student performance is to give formative evaluations/practice tests (Hattie, 2009). If a robot that can score and give detailed feedback on early drafts of essays, we can count these self-scoring essay tests as variations on the paper-based and hand-corrected practice essay-tests of the past.
Conclusion
I’m not arguing for teachers to stop scoring or giving feedback on essays. Rather, I think that a computer-assisted-practice-test approach to writing instruction will help students get the scores and feedback they need to improve without increasing teachers’ workloads.
I therefore remain reasonably optimistic that the Virtual Writing Tutor can, in time, reliably score essays and provide helpful formative feedback to students during the drafting process.
One reason for my optimism is anecdotal but encouraging nonetheless. Frank Bonkowski recently sent me a text message about his experience using the VWT’s automated scoring system with a group of his students earlier in the day. Here is what he wrote.
A super-motivated girl visited the VWT 3x today for feedback on her film analysis essay. She went from 40% to 56% to 88%. She was super happy. Me, too.
Text message from Frank
Me, three.
Works cited
Bridgeman, B. (2004, December). E-rater as a qualitycontrol on human scorers. Presentation in the ETSResearch Colloquium Series, Princeton, NJ.
Farra, N., Somasundaran, S., and Burstein, J., 2015. Scoring persuasive essays using opinions and their targets. In Proceedings of the Tenth Workshop on Innovative Use of NLP for Building Educational Applications, pages 64–74.
Foltz, P. W., Laham, D., & Landauer, T. K. (1999). The Intelligent Essay Assessor: Applications to Educational Technology. Interactive Multimedia Electronic Journal of Computer-Enhanced Learning, 1(2)
Hattie, J. A. C. (2009). Visible learning: A synthesis of over 800 meta-analyses relating to achievement. London, UK: Routledge.
The Way of Chuang-Tzu, by Thomas Merton
Monaghan, W., & Bridgeman, B. (2005). E-Rater as a Quality Control on Human Scores. ETS R&D Connections: Princeton, NJ: ETS.